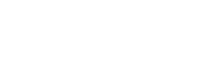
Colloquium: Natural Language Understanding with Limited Training Data
29 April 2019
Natural Language Understanding refers to NLP tasks that require a deep understanding of language, as opposed to shallow, surface-form processing. Most existing research in this area focuses on studying deep learning methods that require large amounts of training data. When labelled training data is not readily available, approaches to utilise knowledge from other sources, such as related tasks or training instances, are key.
In this talk, I will present my recent and ongoing work in the space of learning with limited labelled data in NLU, including research on stance detection, fact checking, information extraction; as well as zero-shot, multi-task and transfer learning.
Speaker: Isabelle Augenstein, PhD, from the University of Copenhagen.
Bio: I am a tenure-track assistant professor at the University of Copenhagen, Department of Computer Science since July 2017 and work in the general areas of Statistical Natural Language Processing and Machine Learning. I head the Copenhagen NLU research group, and am affiliated with the Machine Learning Section. My main research interests are weakly supervised and low-resource learning with applications including information extraction, machine reading and fact checking. Before starting a faculty position, I was a postdoctoral research associate in Sebastian Riedel's UCL Machine Reading group, mainly investigating machine reading from scientific articles. Prior to that, I was a Research Associate in the Sheffield NLP group, a PhD Student in the University of Sheffield Computer Science department, a Research Assistant at AIFB, Karlsruhe Institute of Technology and a Computational Linguistics undergraduate student at the Department of Computational Linguistics, Heidelberg University.
Monday, 29 April 2019, 17:15, Konrad-Zuse Hörsall, Informatikum