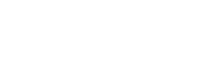
Colloquium: Predictive Modelling in Neuroimaging Data: Opportunities and Challenges
26 November 2024
Colloquium by Dr. Kaustubh Patil, Institute of Neurosciences and Medicine (INM), Forschungszentrum Jülich
Abstract: Machine learning (ML) and artificial intelligence (AI) are increasingly being applied to study how individual differences in the brain can manifest as distinct psychiatric illnesses. These models can help us establish the neural correlates of mental distress and predict individual-level diagnosis, symptoms, trajectories, and treatment responses. In the first part of the talk, I will show several example applications and tools to perform predictive modelling in neuroimaging data. To realize the full potential of these models it is important to recognize their data requirements as well as biases in data and modeling choices that can limit applicability and insights provided by the models. Biases in these models and data can lead to inaccurate and unfair predictions and overlook individual variations, which can have serious consequences for patients, and they can perpetuate and amplify existing health disparities and inequalities. It is crucial to carefully evaluate the risks associated with AI/ML-based modeling such as biases and develop strategies to identify and mitigate them. In the second part, I will discuss risks and challenges associated with such modelling, especially due to biases that can arise from data and modelling choices.
Further information about the speakers can be found here: https://www.fz-juelich.de/profile/patil_k.
The talk is part of the Hamburg Brain School Methods Academy lectures. We organize the present talk as an online event. Credit Points can be obtained as usual as long as you participate for the whole duration of the talk. Please document the participation either on a Laufzettel or for yourself.
Date and time: 26 Nov 2024, 14:00 p.m., zoom webinar (link mailed to list)